Parrobots II
Researching Methods for Extracting System Constraints From Behavioral Data and Applying Them to Building Synthetic Cockatoos
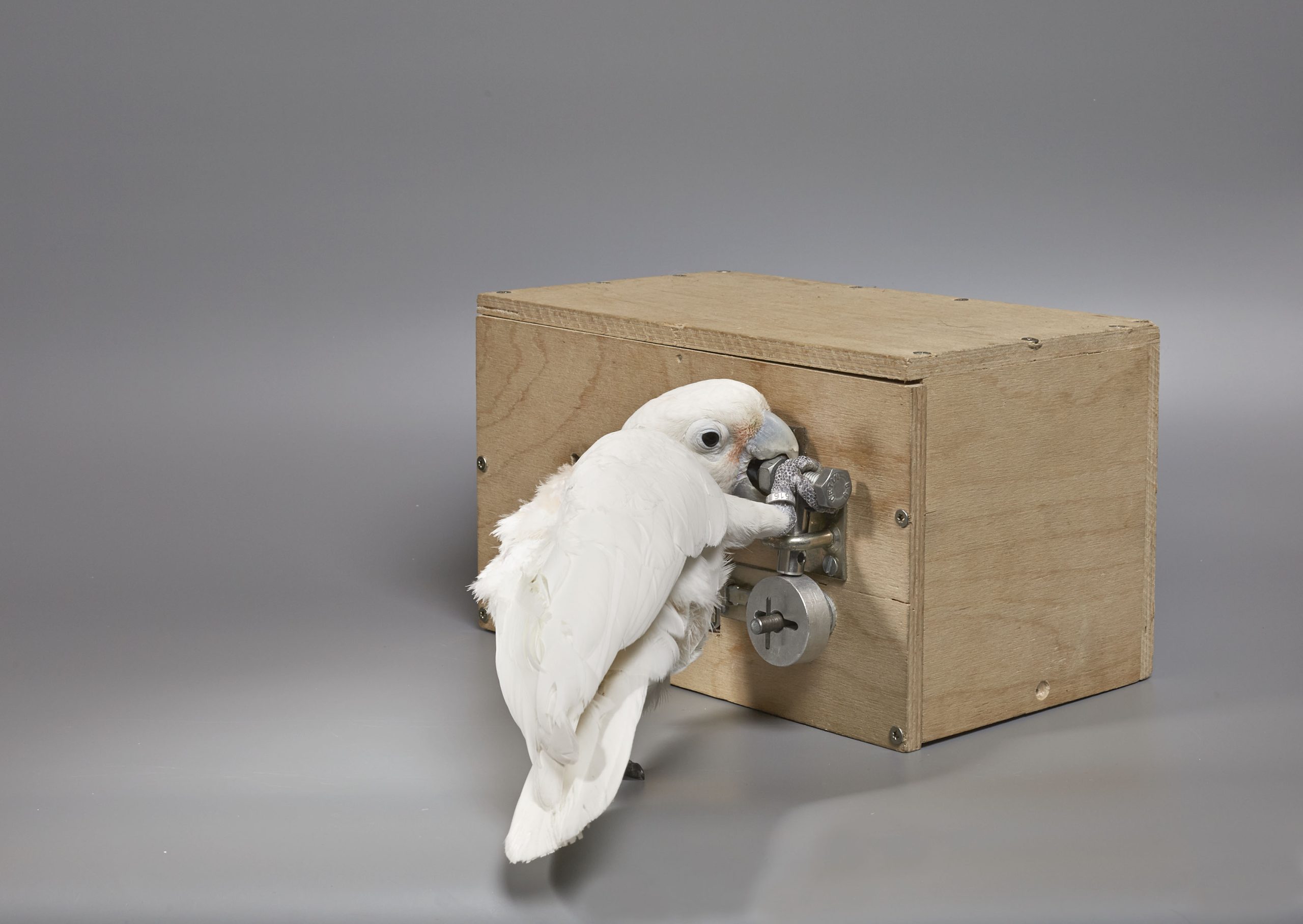
Photo by Goffin Lab, Vienna
When extracting regularities from cockatoo behavior in the context of the lock box scenario (Project 4), we encountered a very fundamental challenge. We found a discrepancy between the number of data we can acquire and the number of data required to conclusively validate a specific behavior-generating mechanism. This is a challenge that needs to be faced by our cluster if we are to extract insights from high-dimensional behavioral observations.
To be able to extract relevant regularites about learning and complex behavior, we must be able to account for the variation caused by individual differences. Only then can we expose in the data the remaining variations that, we hypothesize, describe principles of the underlying mechanistic behavior-generation process. Through exploratory data analysis we will produce categories of properties most helpful in describing individual differences. In Project 4, we already identified several such factors that greatly influence the behavior exhibited by cockatoos when opening a lock box: a) beak- and claw-based manipulation skills, b) motivation to interact with the lockbox, and c) understanding of the mechanism (operationally defined as being capable of innovative actions appropriate for the state of the problem). If we want to understand how the bird progresses to stage c), we have to first quantify and extract from the data knowledge about a) and b). These distinctions have become sharper through our previous research: we found that an animal can improve performance without increasing understanding, and that conversely, it may understand the problem but fail to solve it through lack of mechanical skill or motivation.
The methods proposed so far will take complex, high-dimensional behavioral data and through an interactive process of analysis (and possibly also experiment) inspired by the results of Project 4, produce: 1. Accounting for Individual Differences: A number of factors that explain variations in the observed behaviors, i.e., explain aspects of individual differences (e.g. motivation, interactive abilities, knowledge of the problem, prior experiences); 2. Extracting Constraints on Mechanistic Explanations From Behavioral Data: A set of constraints on mechanistic explanations of the observed behavior that is self-consistent and consistent with the data.
The models obtained in the previous project component will be implemented on our synthetic test platforms (of course, they won’t really be like cockatoos). We will proceed from simple to complex. We will start with simple mathematical models, proceed to state-machine-based simulations, then possibly to physical simulations of robotic agents, and finally to our real-world robotic mobile manipulation platform. The latter is identical to the platform used in the escape room scenario of example behavior 1. This ensures that the synthetic output of this project is immediately integratable into the escape room demonstrator. In accordance with the A/S loop, we will empirically study the behavior of the robotic artifact. We hope to derive further insights for the Exploratory and Hypothesis-Driven Data Analysis described above. We believe that this loop with the behavioral data (rather than with new cockatoo experiments) will be a very efficient way of making progress. Nevertheless, we anticipate performing additional cockatoo experiments, once our attained understanding clearly justifies this.