Mouse lock box 2
Intelligent Problem-Solving in Mice
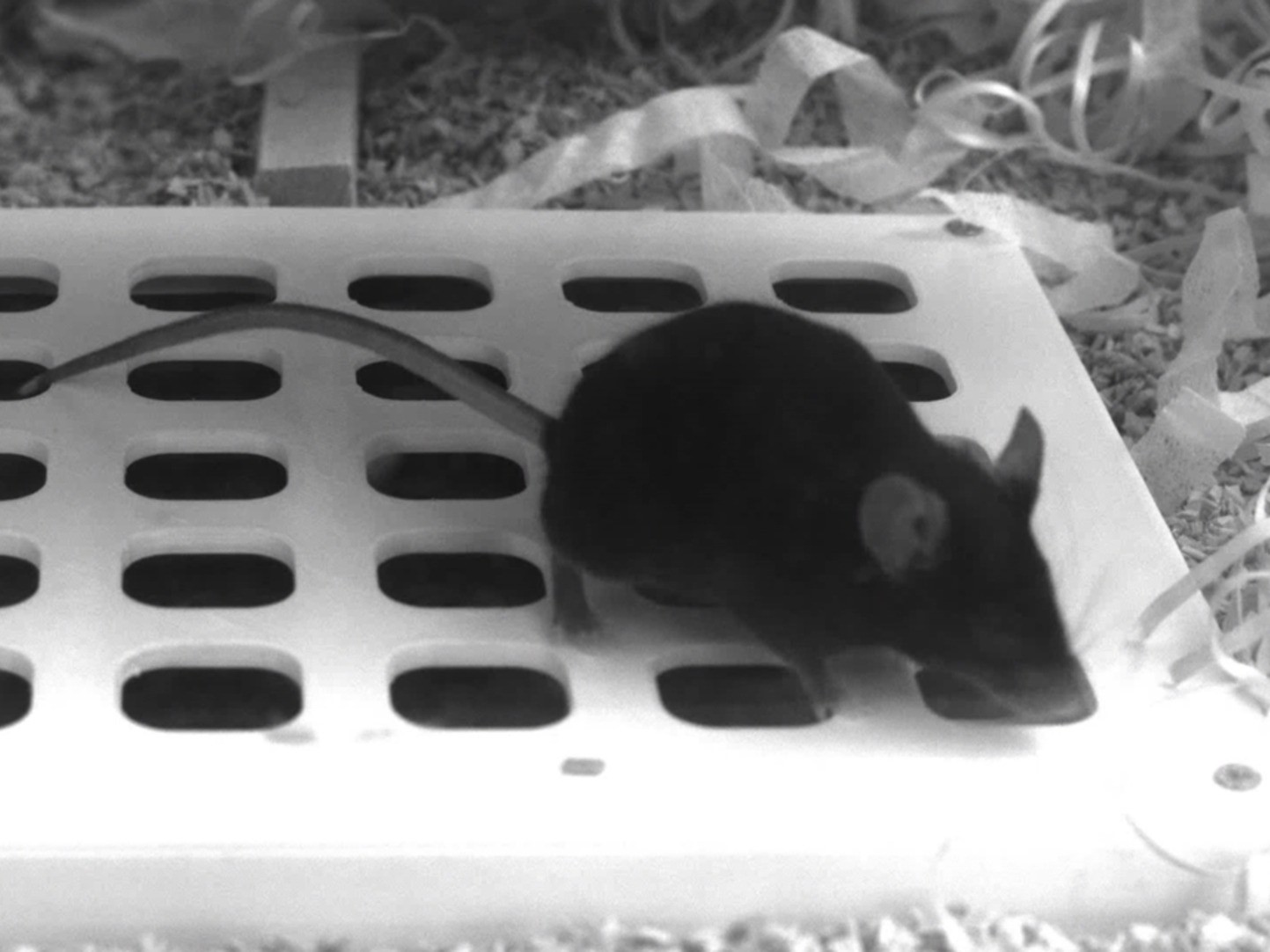
©SCIoI/Katharina Hohlbaum, Arianna Novati
This project builds on our previous Project 03, in which we investigated intelligent problem-solving in mice. We developed two lockbox sets for mice, each consisting of four “single mechanism locks” and a “combined lock box”. The combined lock box unites all four single mechanisms, which block each other. Over the course of the project, we could demonstrate that mice are able to solve different types of lock boxes, that their performance improved over time, and that they even appeared to enjoy the task. We acquired a large body of video material (>200h of video from 12 mice) and established a tracking solution that allows an automated pose estimation of the mice, as well as a deep learning-based solution to estimate the emotional state of the animal from facial expressions (Andresen et al., 2020). Yet, the project also leaves us with a host of unexplored data and a variety of natural follow-up questions, which we aim to address in the present project.
Firstly, we need to sharpen our data analysis tools in order to maximize the use of the video material. To this end, we will derive 3-dimensional “skeletal” models of the mice for as many video frames as possible, using machine learning to overcome problems of tracking failures and visual occlusions. Secondly, we will use this to understand how and on which level of abstraction the mice solve the problem. Do mice acquire an abstract schema of the task (Tse et al., 2007) that allows them to solve a qualitatively different lock box? Do they approach the combined lock box in a planful way upon the first encounter or do they simply explore all lock box parts randomly? On the finer level of motor control: Do the mice simply learn to smartly “rattle” the lock box in an exploratory fashion – which would allow them to solve a mildly modified lock box – or do they learn an idiosyncratic solution that exploits the physical details of the lock box part? We will address these questions by i) performing extensive data analyses and modeling of existing and new data – with a focus on the substantial behavioral variability within and across mice, and by ii) using a reconfigurable version of the lock box – “a multiple access lock box” – to study if mice can flexibly generalize to related lock box tasks. Finally, we will try to identify the control laws that govern the movements of the mice, to synthesize artificial trajectories of mice solving different parts of the lock box.