Big mouse data
Designing “natural” tasks from a mouse-centric perspective
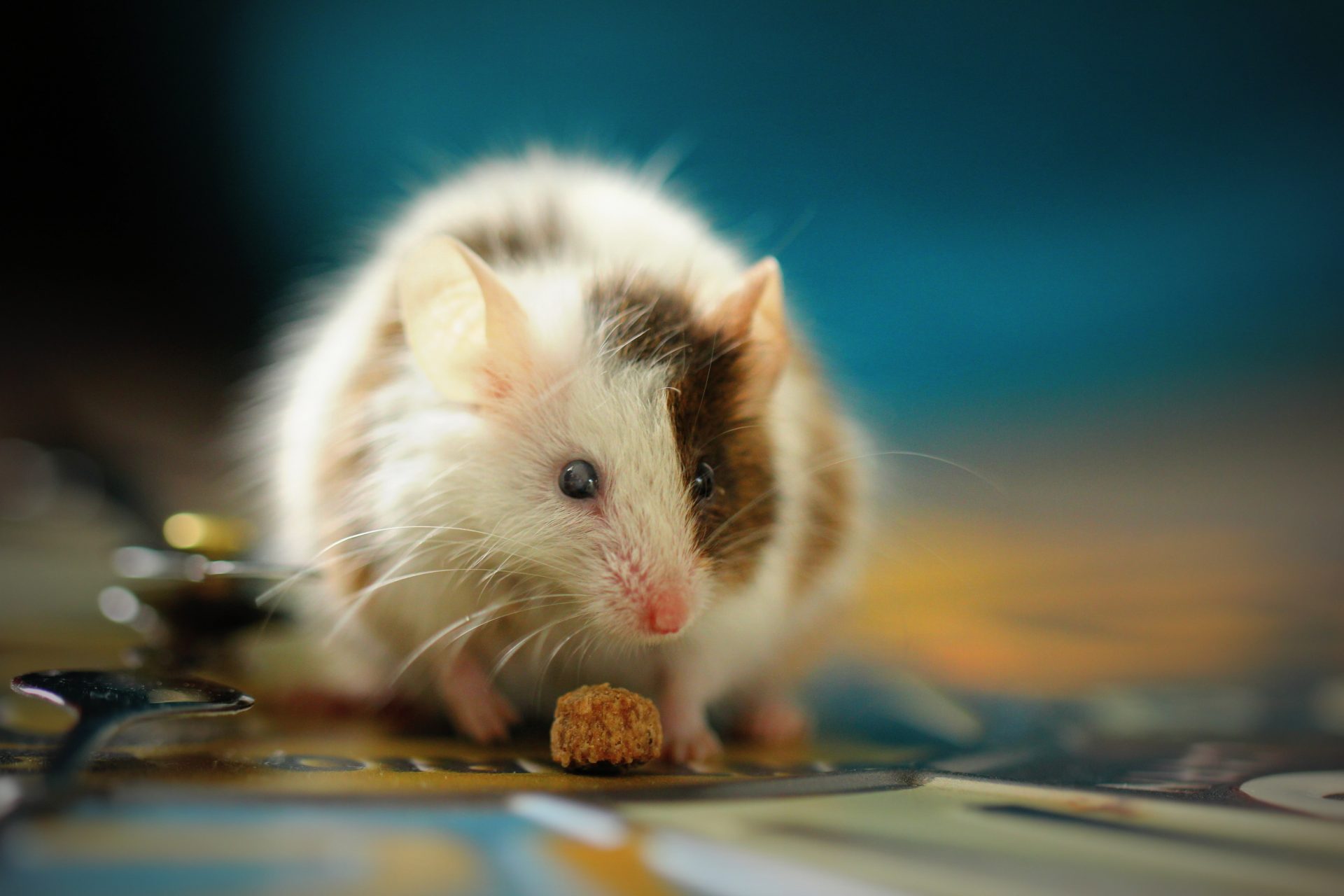
Photo by Sandy Millar on Unsplash
A basic tenet of SCIoI is that intelligent behaviour is tightly tied to the ecological niche in which it is performed. Yet, the behavioural assays that are used to study animal behaviour in the laboratory are typically artificial and far removed from natural behaviour. It is therefore not surprising that it is often hard to predict how successfully a newly designed task is learned by a given species or animal.
The goal of the present project is to develop a framework for designing ecologically valid learning tasks by adopting an “animal-centric” view. To this end, we will harness the technology we established in our current SCIoI projects to track the behaviour of mice in their home environment over long times, establishing a “big data”-base of unconstrained mouse behaviour. We will then use this database to build models for individual animals, in the form of probabilistic models for sequences of behavioural primitives, including ultrasonic vocalizations. These models will allow us to quantify the probability that any given sequence is performed by a given mouse during its natural behaviour. Our hypothesis is that this probability is a proxy for how “natural” a particular behaviour is to a given animal, and that this in turn predicts how easily a particular behaviour is learned. Just like humans that easily learn math or languages tend to specialize in these skills and overrepresent them in their behavioural repertoire. We will test this hypothesis by identifying behavioural sequences (“tasks”) that reflect the individual preferences of different mice and then perform operant conditioning to study how easily these tasks are learned by different mice.
This will allow us to address a variety of questions. Are “natural” tasks indeed more easily learned than artificial ones? How variable are the individual learning skills of mice? Are those tasks that are most “natural” to a given animal the easiest to learn or is there an “optimal level of challenge”? How do we best design curricula to teach a given animal a given skill? Can we design a variant of an escape room/lock-box that is as natural to a mouse as locks and bolts are to humans? This project would contribute to a rapidly increasing trend in behavioural sciences to analyse complex natural behaviour by combining advanced tracking, sensor-fusion, data analysis, machine learning and modelling methods. The long-term goal is to initiate a paradigm shift towards an ecologically grounded and individualised approach to animal learning and understanding intelligence that may ultimately allow a bridge to human educational science.