Learning of intelligent swarm behavior
When is it ecologically beneficial to act as a collective, or to develop diversity within a swarm? When is it best to act alone?
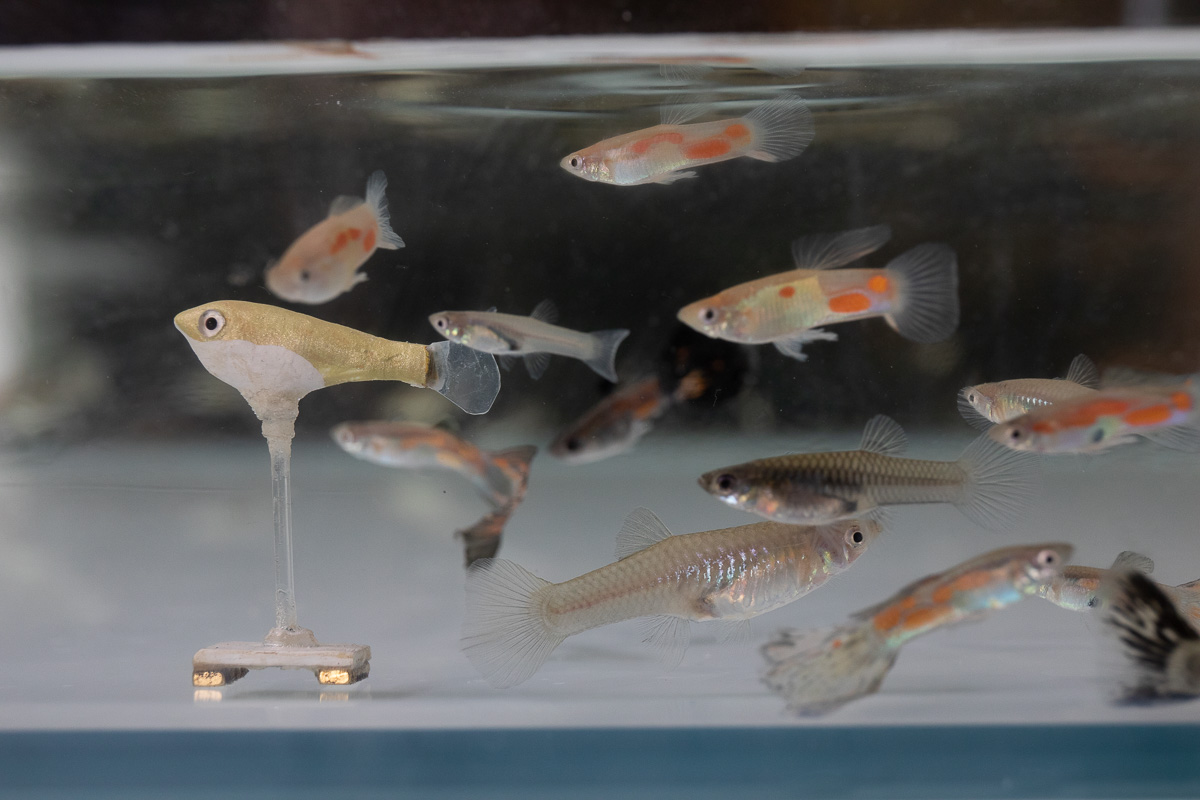
©SCIoI
The proposed project will focus on the following four main objectives: In a first step, we will focus on developing analytical methods to identify heuristics of anticipation in live fish that interact with a biomimetic robotic fish (Robofish) and vary also in social responsiveness (objective a). In a second step, we will create situations in which live fish have to cooperate or compete with Robofish in order to achieve a goal effectively. This will allow us to estimate costs and benefits associated with anticipatory strategies (objective b). The aim of our experimental data is to identify cognitive heuristics that play a role in anticipation in interacting pairs that will then be used to develop a ubiquitous synthetic behavior of anticipation when social responsiveness in interaction partners varies (objective c). In order to evaluate this synthetic behavior, it will be implemented into Robofish as well as in humanoid robots and then tested in situations involving either robot-only pairs (robot-robot) or, in case of Robofish, also pairs with one live agent (fish-robot) (objective d).