Observational learning developing humans and artificial agents
Observational learning in children and robots
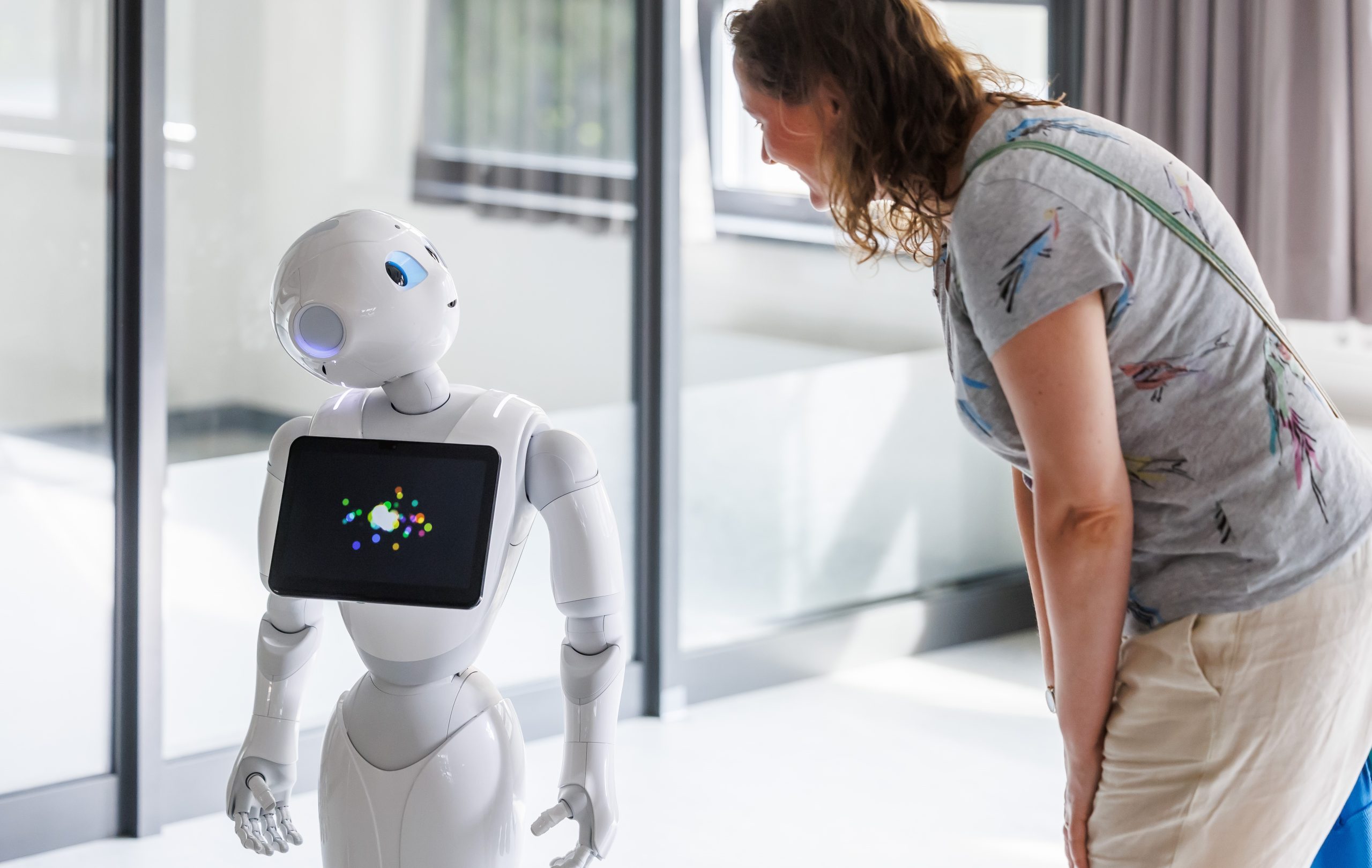
Observational learning (learning from actions and outcomes of others, short OL) is particularly relevant for the offspring of intelligent species as it allows a rapid acquisition of new behavior (without having the potential costs of individual trial-and-error learning, e.g., about potentially toxic food or using sharp tools without injuries). Furthermore, it is a way of efficiently transmitting successfully learned behavior across generations, permitting cultural evolution. The questions how and from whom developing individuals learn via OL are of interest across different disciplines, including psychology, cognitive neuroscience, and robotics (e.g., Rodriguez Buritica et al., 2016; Chevalère et al., 2022). Merging these different perspectives helps to develop synthetic models of how others’ information gets integrated during learning, which can fuel applications in robotics (e.g. in the educational sector and in HRl) and increase our understanding of OL in humans and the role of cultural evolution.
ln this cross-disciplinary project, we aim to address these questions using a foraging-task in virtual reality (VR) that can be used both for behavioral experiments with developing humans and artificial agents. We investigate how children integrate actions (i.e., reaching and selecting food items) and outcomes of others. Within this foraging task we track how observed information is integrated in children (objective 1) and how these dynamics can be captured by computational models (objective 2). We implement the extracted OL dynamics in artificial agents (objective 3), and compare OL in children and artificial agents with different computational models (objective 4). ln future projects, we will study from whom children and artificial agents learn by investigating how a-priory observed characteristics of others (such as trustworthiness), particularly important in more complex foraging environments, influences OL.