Alan Akbik
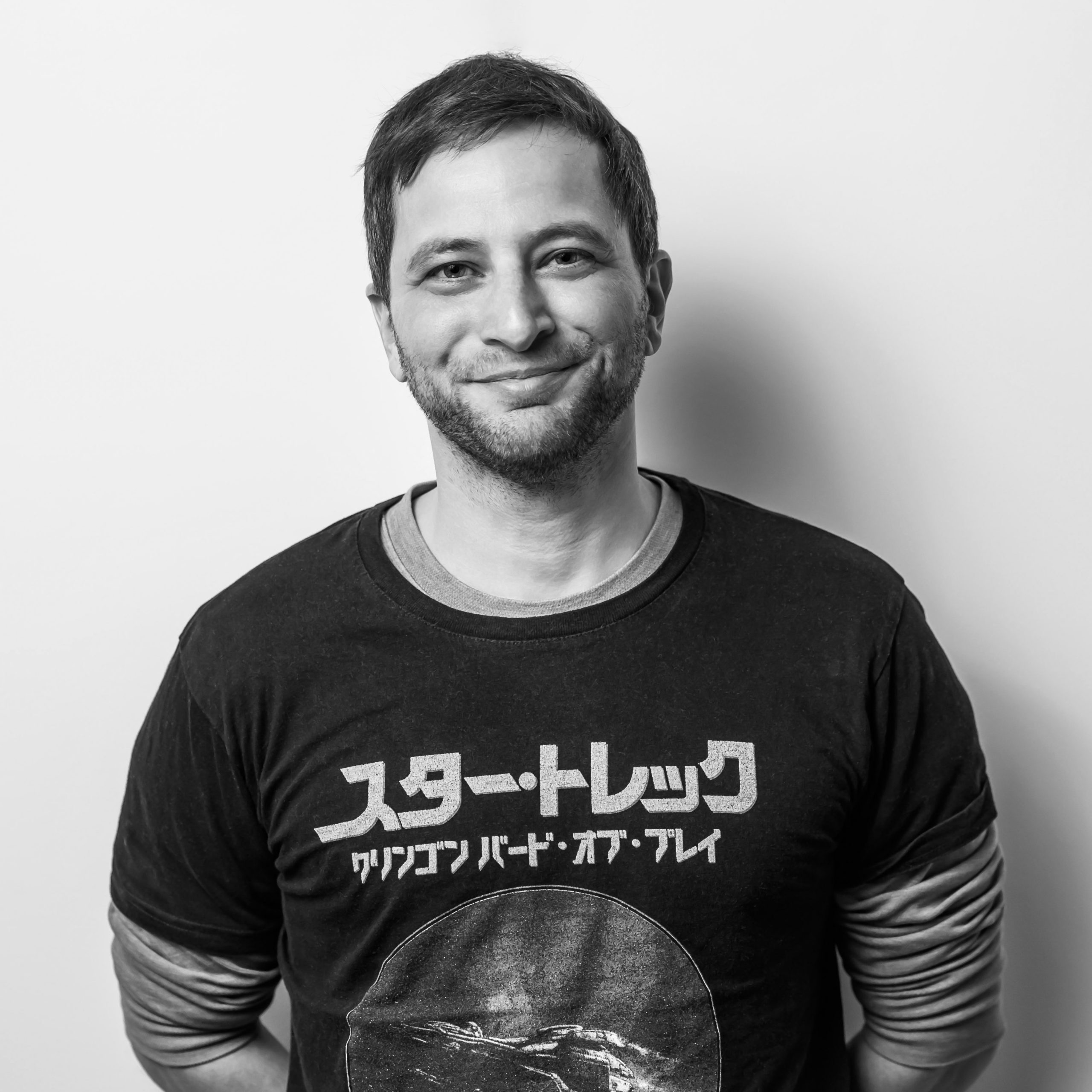
Photo: SCIoI
Alan focuses on research in machine learning (ML) and natural language processing (NLP), with the goal of giving machines the ability to understand and use human language. This spans research topics such as neural language modeling, sample-efficient learning and semantic parsing, as well as application areas in large-scale text analytics. Together with his group and the open source community, he develops the NLP framework Flair (https//github.com/flairNLP/flair) that allows anyone to use state-of-the-art NLP methods in their research or applications. At SCIoI, Alan works at Project A002, Project 44, and Project 45.
Projects
Alan Akbik is member of:
• Project 44: Efficient model learning from data with partially incorrect labels
• Project 45: Modeling neurogenesis for continuous learning
Publications +
EACL’s Outstanding Paper Award (2023)
Emmy Noether Grant (2021)
Thursday Morning Talk -
Alan Akbik (SCIoI): Automatically Understanding Human Language: Challenges and Applications